Eliminate AI bias through accurate data labeling
The elimination of bias in AI hinges on the quality of labeled data, expert-in-the-loop solutions, and the geographic diversity of labeling teams
The scepter of AI bias
The terms AI and bias taken together might seem contradictory owing to the belief that technologies like AI are predicated on combating human-related prejudices. But AI algorithms are only as good, or as bad, as the data that engineers feed them. If engineers harbor prejudices and discriminatory worldviews, the algorithm can inherit them and churn out corresponding output — a phenomenon known widely as AI bias. Even rogue data can fuel AI bias because of being incomplete and uncontextualized; if there is room for several interpretations, there is no guarantee that algorithms will provide a desirable output.
This raises the question of how to approach AI bias in light of increasing digitalization and proliferation in AI-ML applications. According to Gartner research, if the issue persists, by 2030, 85% of AI projects will provide false results. Considering AI is permeating business and industry — even critical areas like defense, retail, and healthcare — the consequences of biased results could be disastrous. In fact, there are early tell-tale signs of the potential pitfalls. Amazon once commissioned a resume-vetting AI project, whose algorithm was built on ten-year recruitment data. Soon, the company learned that the AI recruiting tool was dismissing female candidates. It emerged that the algorithm was merely responding to historical hiring decisions, which favored male candidates over females. These revelations later led to the project being decommissioned.
The consequences of bias can prove disastrous for businesses
To put things into perspective, Amazon employs 1.3 million people worldwide! And gender discrimination is only one among the 180 human biases classified by psychologists. So, in a hyper-digital industry like e-commerce, AI bias could exacerbate existing inequalities, widen the digital divide, and perpetuate discrimination. If the facial recognition function, for example, is fed more images of light-skinned faces than dark-skinned ones, the system is bound to provide undesirable suggestions and services to people with darker skin tones. It is to this effect that AI adopters must emphasize high-quality labeled data and human-in-the-loop solutions.
The anatomy of bias in stages
According to a data scientist, AI bias can occur at various stages of an AI-ML project, from design to later testing and monitoring. Commonly, in the design and formulation stage, the project is prone to sampling bias, where only a population subset or an outgroup is sampled, making the data skewed towards them.
Gaps in the data could hamper hyper-personalization and segmentation in eCommerce
In the pre-processing and exploration stage that follows, underrepresentation of a minority dataset, use of obsolete labeling technology, and incorrect annotation of a dataset can lead to recall bias. At times, faulty measurements during this stage lead to disparities between training and real-world data. Engineers are also prone to exclusion bias, where they may omit pertinent data during the cleaning, thinking it is irrelevant. In a consumer-facing industry like e-commerce, such data gaps hamper hyper-personalization and segmentation.
The ensuing model development stage is a source of considerable AI biases. The nuances can be listed as follows.
- Time-interval bias: A prejudiced selection of a dataset pertaining to a certain time frame, betraying other vital periods.
- Survivorship bias: Instances where the collated data only pertains to surviving points like analyzing leakages in packaging by checking only cleared orders ignoring ones that were discarded due to leakages.
- Omitted variable bias: Exclusion of one or more critical features from an AI-ML model.
- Confounding bias: The result of a confounder — a variable that influences both response and predictor variables — in the model.
Subsequently, in the model interpretation stage, AI biases are perpetuated by common phenomena like confirmation bias, which is a tendency to favor data that confirms the engineer’s entrenched beliefs. Such engineers often assign higher weightage to favorable predictors, thereby betraying other determinants. The biases at this stage also extend to funding, wherein decisions favor financial sponsors, select investors, etc., and cause-effect bias, which equates correlation with causation.
Bias must be proactively combated at every stage of the AI-ML pipeline
Finally, in the validation and monitoring phase, AI projects grapple with high or low statistical bias due to either the lack of features in the training dataset or a highly-flexible model’s inability to generalize. Algorithms can characterize both underfitting and overfitting. Also, at times, there could be an overlap between training and test data — in which case, the impact could be quite evident in the output, enabling redressal.
Identifying and addressing AI bias
Human biases are ubiquitous and have existed since the dawn of time. One of the underlying rationales of technologies like AI is to remediate such human errors. With AI being as far as we have reached to emulating human capabilities minus the shortcomings. So, if its efficiency hinges on unbiased labeled data, we have a collective obligation to facilitate it.
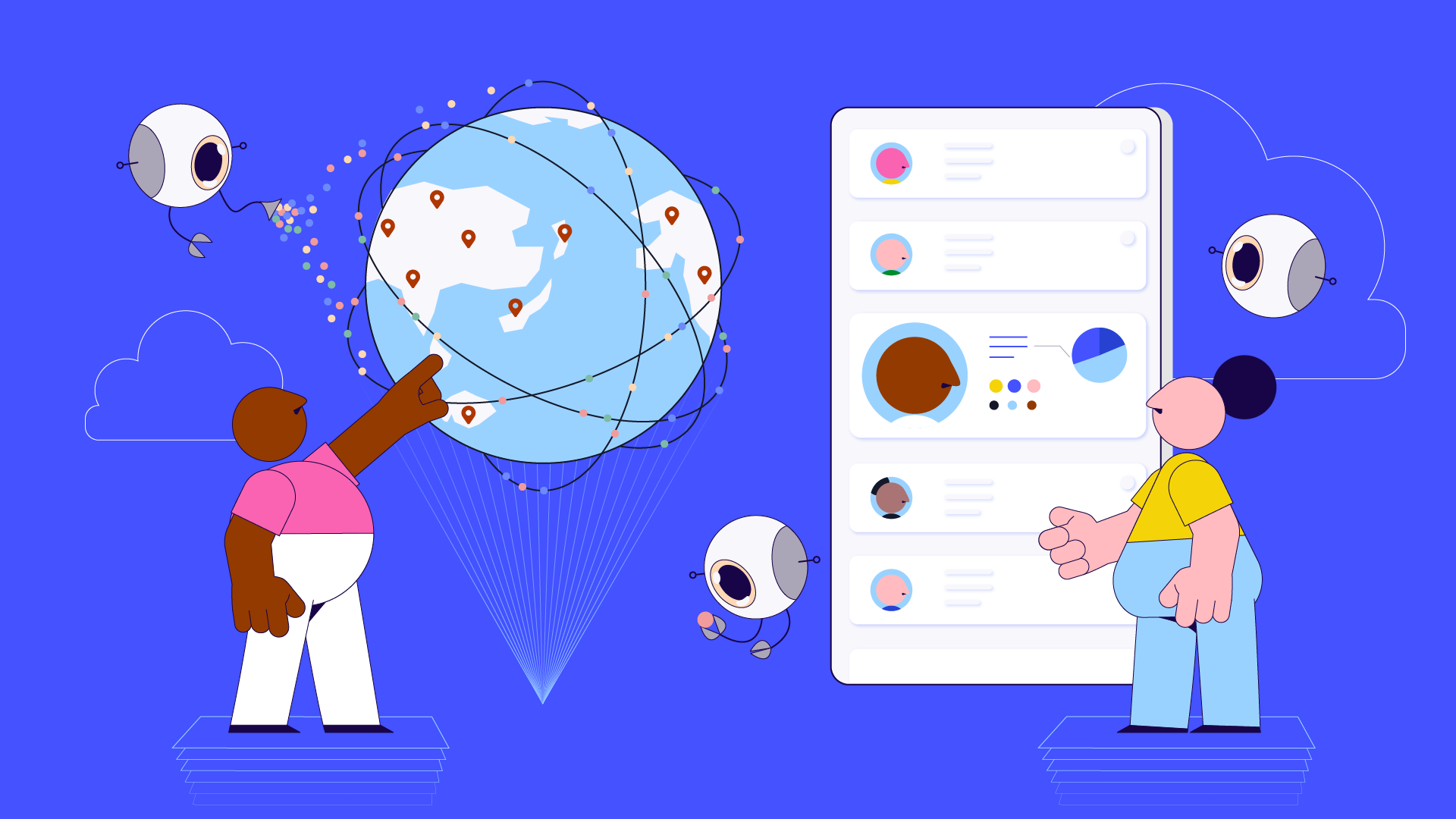
Human-in-the-loop solutions and geographically distributed vendors
The ongoing AI proliferation means that the scope of data will increase too, requiring accurate data labeling. The foremost priority is to then contextualize the data, focusing especially on aspects that are susceptible to biases. Contextualization also requires human/expert-in-the-loop solutions, which comprise algorithm-driven processes that are double-checked by humans. Without a human-in-the-loop provision, biases will snowball and go unchecked, leading to more complexities.
Eclectic data procurement strategies
Inclusion is a proven formula to combat biases. Therefore, it’s advisable to have inclusive workforces and vendors, with representations from across the globe, race, ethnicity, and gender. Even data procurement must be eclectic.
To solve the problem, one must first fully understand it. So, stakeholders must greatly emphasize bias research, hoping to understand causal factors and plausible solutions. Industry leaders, in particular, must focus on establishing gold standards for labeling sets. This could include detailed directives on collating, sampling, and pre-processing of data. Most importantly, leaders have a pivotal role to play in raising awareness of existing biases and their manifestations.
No-bias data labeling: The Taskmonk way
As a purpose-built e-commerce data labeling platform, Taskmonk has crafted a niche for itself over the years. Its growth is fueled by an eight-fold increase in AI investments by e-tailers. As investments increased and applications expanded, the need for no-bias labeled data only amplified, supercharging Taskmonk’s growth.
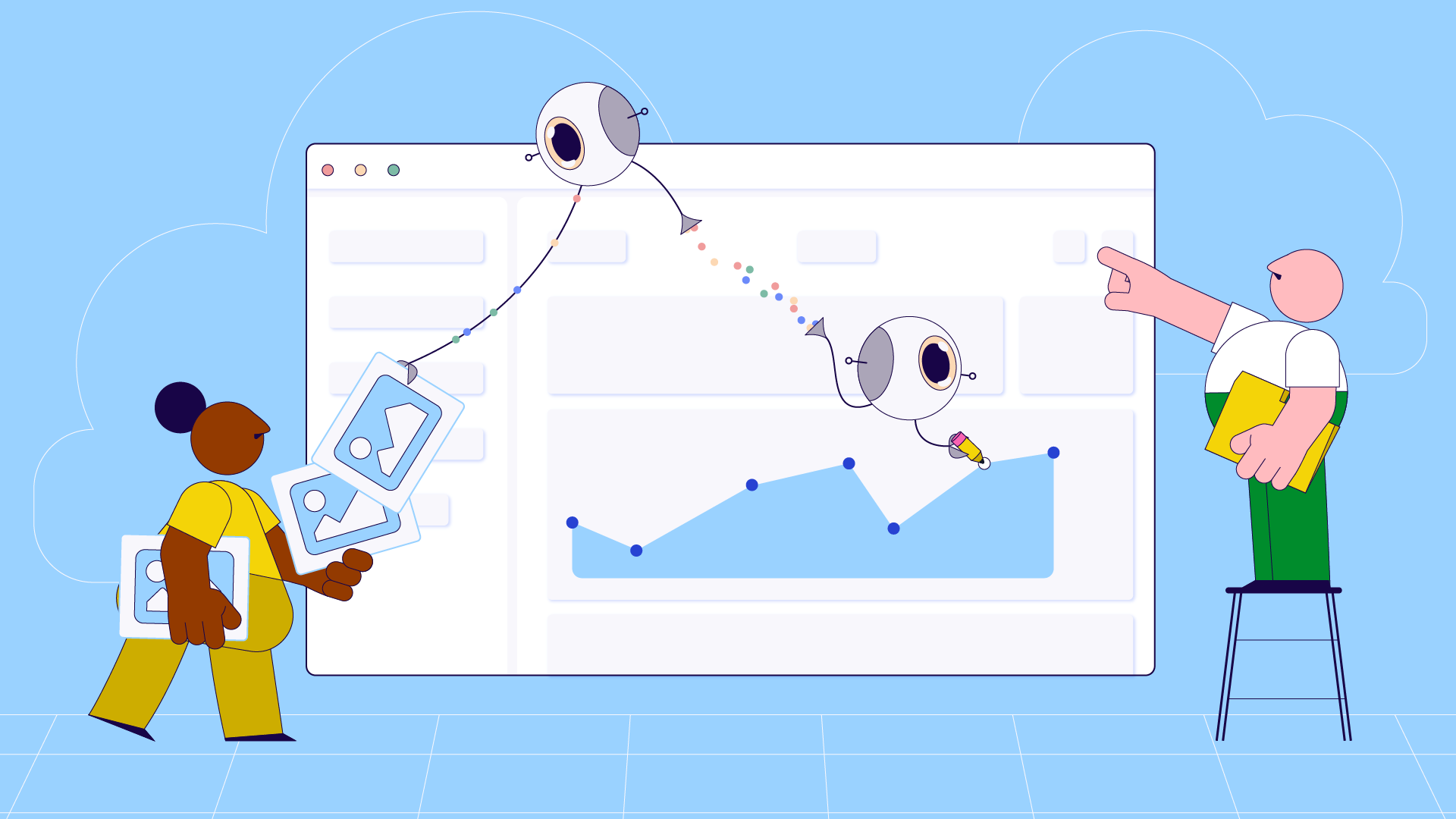
Hybrid workflows and geographically diverse team of annotators
The company’s success is also owed to its multi-dimensional approach, implemented by a global team of annotators who bring diversified perspectives, linguistic capabilities, and understanding of local sensibilities to the table. Thanks to its hybrid workflows, Taskmonk successfully operates with diverse in-office and remote teams.
Golden datasets and pre-processing algorithms
Taskmonk’s use of golden data sets (clean, integrated data sets), its creation of pre-processing models that prioritize combating bias, and its emphasis on constant monitoring, reporting, and analytics, too, constitute its best-in-class services. The constant monitoring, in particular, is an important value proposition because, as data availability increases, the stakes will only go up. Most importantly, Taskmonk understands AI bias for what it truly is — a reflection of human behavior. So, it’s not in the least bit surprising that AI bias is a widespread phenomenon like any form of real-world discrimination and prejudice. However, unlike humans, AI can deftly rid itself of biases — making the pursuit of zero-bias AI all the more gratifying for a service-driven company like Taskmonk.
- https://www.gartner.com/en/newsroom/press-releases/2018-02-13-gartner-says-nearly-half-of-cios-are-planning-to-deploy-artificial-intelligence
- https://www.reuters.com/article/us-amazon-com-jobs-automation-insight/amazon-scraps-secret-ai-recruiting-tool-that-showed-bias-against-women-idUSKCN1MK08G
- https://towardsdatascience.com/eliminating-ai-bias-5b8462a84779
- https://docsend.com/view/yhna3nrri3dgkxyw